Το TinyML ταξινομεί και ιεραρχεί τους ασθενείς του Covid-19 στα Τμήματα Έκτακτης Ανάγκης
Παρά το γεγονός ότι βρισκόμαστε στη μέση ενός σημαντικού μετασχηματισμού όσον αφορά τον τρόπο παραγωγής και διανομής προϊόντων και υπηρεσιών, γνωστού ως Βιομηχανία 4.0, η Υγεία 4.0 βρίσκεται ακόμη στα σπάργανα. Ωστόσο, οι νέες τεχνολογίες και τεχνικές IoT κάνουν τη διαφορά με στόχο να αλλάξουν τον τρόπο παροχής υγειονομικής περίθαλψης. Το πρόσφατο ξέσπασμα του Covid-19 ανέδειξε τις αδυναμίες των συστημάτων υγειονομικής περίθαλψης σε όλο τον κόσμο και αποκάλυψε την κλινική ανάγκη για νέες καινοτόμες λύσεις IoT.
Προκειμένου να περιοριστεί η εξάπλωση του Covid-19, απαιτούνται ορισμένα μέτρα για την ανακούφιση των οργανισμών δημόσιας υγείας, συμπεριλαμβανομένων των νοσοκομείων αναφοράς. Ένα από τα σημαντικότερα είναι η μείωση του υπερπληθυσμού των Τμημάτων Επειγόντων Περιστατικών (ΤΕΠ), η ελαχιστοποίηση του χρόνου αναμονής των ύποπτων περιστατικών και η αύξηση της ασφάλειας εντός του νοσοκομείου. Ο πυρετός και η δύσπνοια θεωρούνται δύο από τα κύρια συμπτώματα των ασθενών που έχουν μολυνθεί από το Covid-19. Και τα δύο συμπτώματα μπορούν να παρακολουθηθούν αντικειμενικά με τη μέτρηση ζωτικών σημείων, όπως η θερμοκρασία του σώματος, ο ρυθμός αναπνοής ή/και τα επίπεδα κορεσμού οξυγόνου. Η συνεχής παρακολούθηση αυτών των ζωτικών σημείων δεν θεωρείται επί του παρόντος ως επιλογή που προσφέρεται από τα ΤΕΠ, εκτός εάν ο ασθενής εισάγεται στη ΜΕΘ. Αντ' αυτού, μπορεί να γίνονται σποραδικοί έλεγχοι ανάλογα με τη διαθεσιμότητα των ανθρώπινων πόρων (συνήθως περιορισμένων και υπό τεράστια πίεση). Επιπλέον, οι διαθέσιμες στο εμπόριο ιατρικές συσκευές δεν είναι πάντα φορητές, ενώ απαιτούνται περισσότερες από μία συσκευές για την παροχή του πλήρους φάσματος των απαιτούμενων ζωτικών σημείων.
Exploiting edge computing and advanced digital signal processing techniques, Vidavo designed and developed a wrist-wearable device able to continuously extract heart rate, blood oxygen saturation, body temperature and evaluates patients’ respiration. A significant novelty of the proposed solution is the use ML algorithms for respiration evaluation. Specifically, our team has designed and developed a machine learning model using Neural Networks (NNs) that can be run locally on the wearable device in order to avoid transmitting raw data over a radio channel to expensive, power hungry and vulnerable cloud infrastructures, ensuring data privacy, security and minimizing latency. The NN identifies three main patterns regarding respiration and deploys them on the wearable device in order to access and classify respiration patterns locally and offline highlighting the advantages of edge computing. The NN was designed and build using Edge Impulse, a user-friendly web application in order to execute the whole toolchain of a neural network, from raw data acquisition and extracting features to training models and deploy them on embedded devices.
The wearable device has been already interconnected with Vida24©, which is an integrated care solution, connecting smart medical devices, patients and medical experts already in use by thousands of EU citizens. Vida24© is certified CE0653 Class IIa medical software according to the EU Medical Devices Regulation (MDR 2017/745) and GDPR compliant.
The wrist-wearable device supports common communication protocols, thus being able to transfer processed data to other third-party systems and apps through an API. A mobile application for the ED personnel is used for real-time detection of health deterioration and enables highlighting the most critical Covid-19 cases, thus reducing clinical decision-making and patient waiting time and allowing for more efficient triage. Finally, recorded vital signs might act as a complementary source of information to the results of the clinical examination to make a final diagnosis of patients.
Working with our partners from Medical Physics lab from Medical School of Aristotle University of Thessaloniki, the proposed solution has been set up and piloted in the ED of AHEPA hospital in Thessaloniki, Greece, where suspicious Covid-19 cases are referred to from the Region of Central Macedonia.
The paper was published on IEEE GLOBECOM 2020 Special Workshop on Communications and Networking Technologies for Responding to COVID-19 in Taipei, Taiwan on December 2020. You can download the paper from IEEEXplore®.
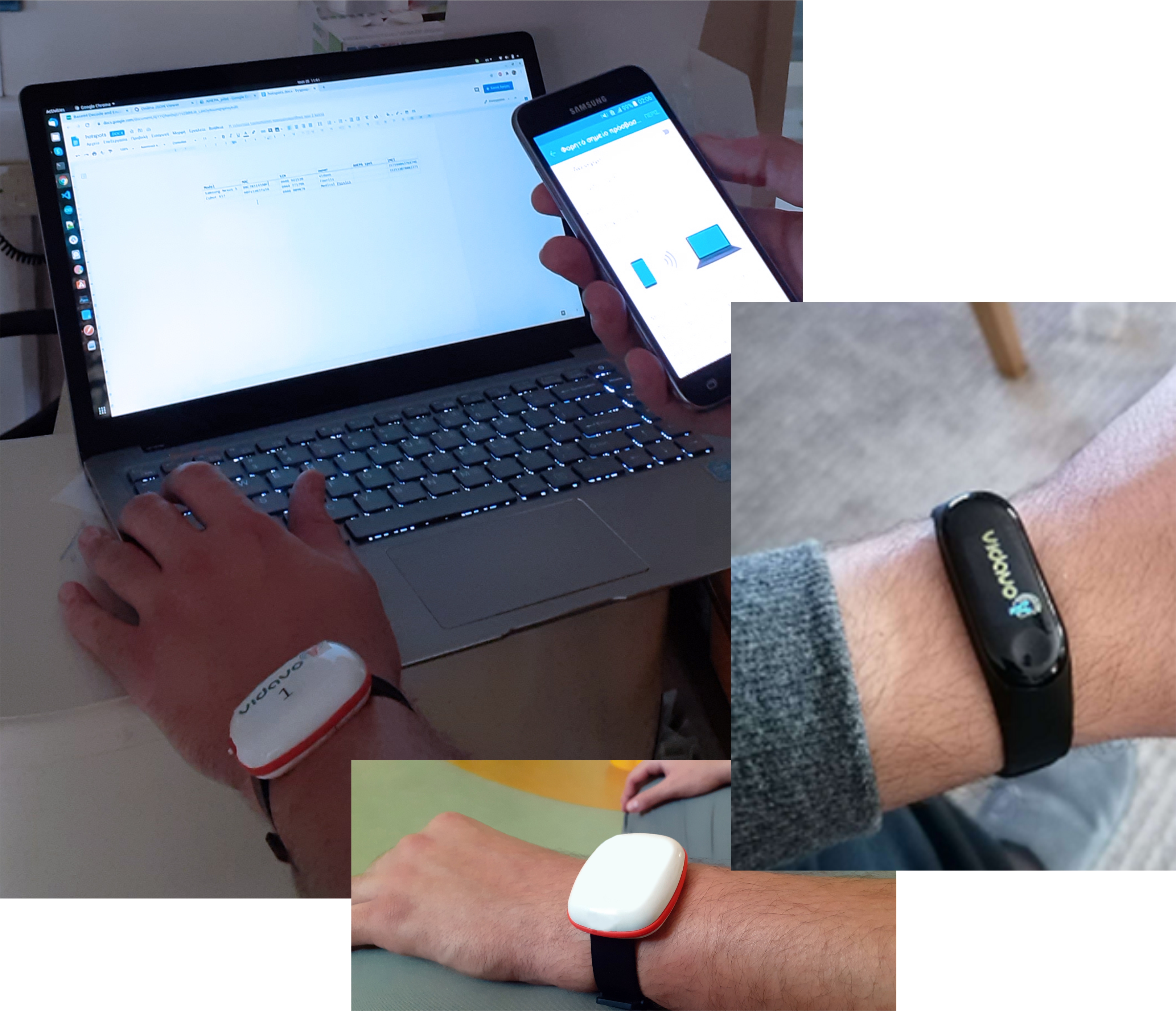
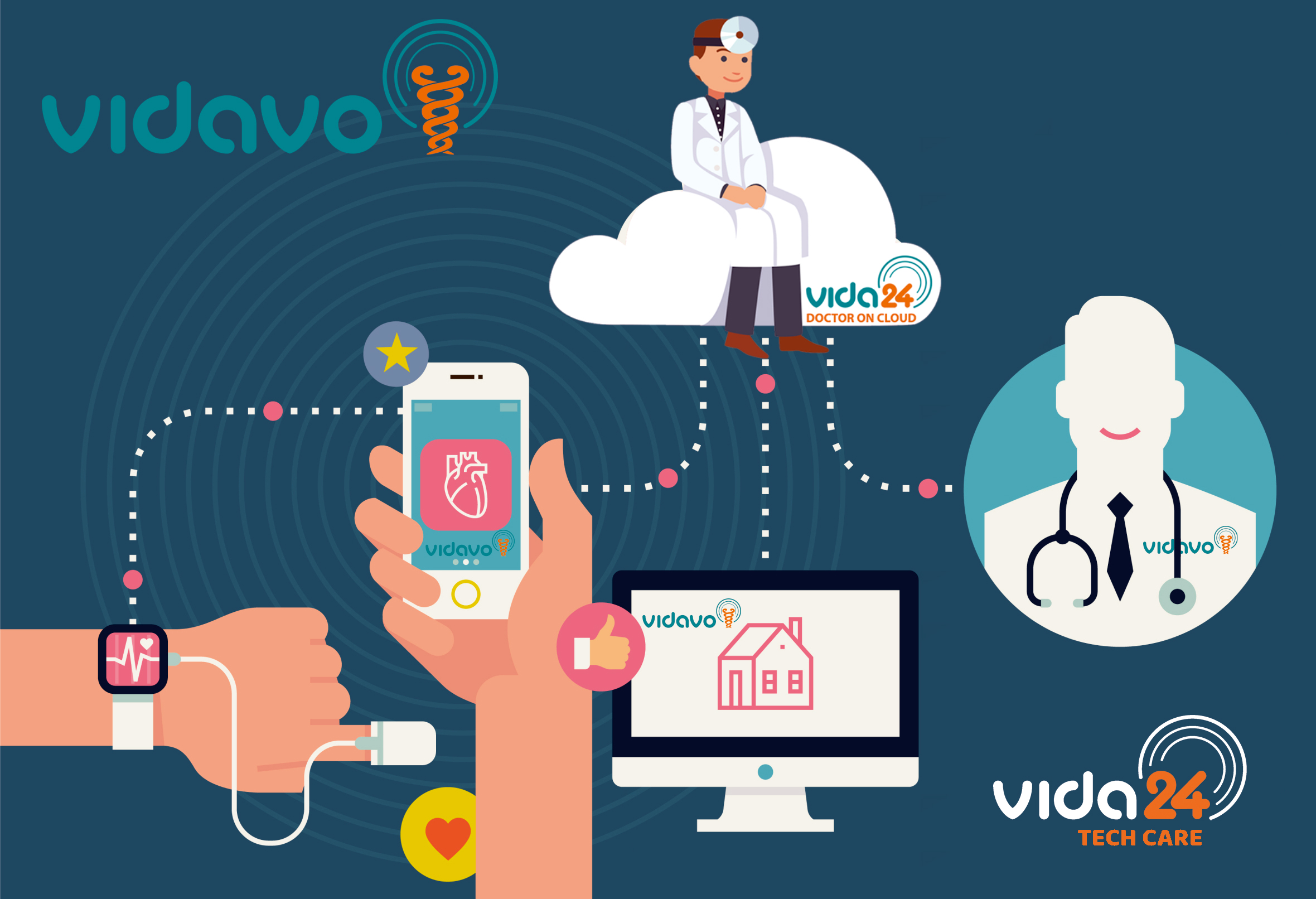